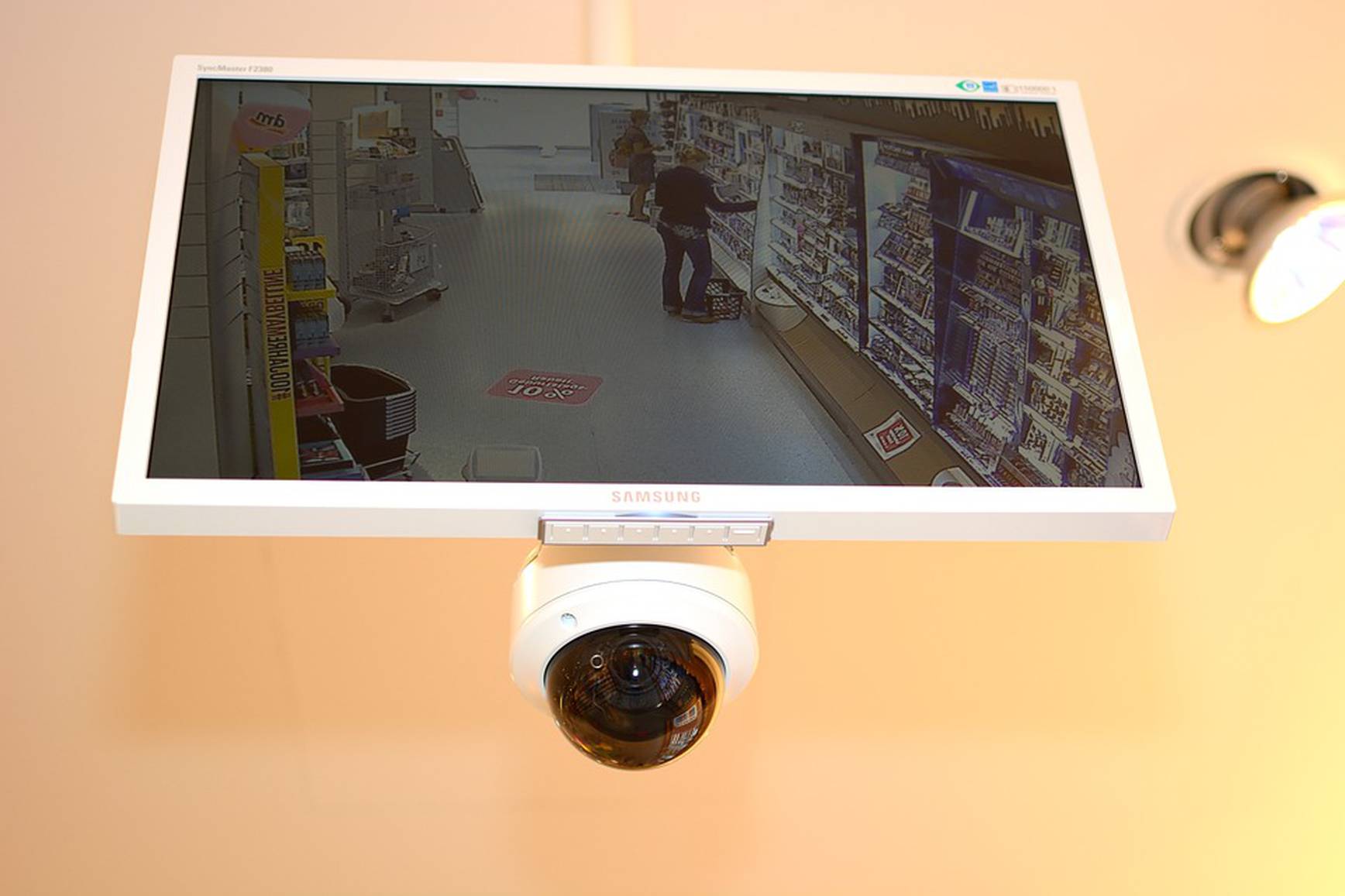
BY PROFESSOR YI YANG
Many of us would be familiar with the following scenario in films: police notice a criminal in a black jacket, dark pants and black shoes in a surveillance camera near the airport, which matches the pictures and text descriptions in a database collected previously. The police then take actions to track down the criminal. This is in fact an example how video analysis techniques help law enforcement. Beyond the films, Australian researchers from the University of Technology Sydney are collaborating with D2D CRC in creating effective big video data management tools and knowledge for law enforcement.
The D2D CRC stream Semantic Indexing of Large Scale Video Archives, part of the Apostle project, aims at tackling such problems by analysing unstructured videos, e.g. surveillance, and turning them into structured data stored in a database. Deep learning methods, which have been leading the revolution in Artificial Intelligence, are the key to this process. In ways similar to the human visual stream, deep learning architectures are featured by a tremendous number of learnable parameters and are believed to be more effective with increasingly deeper models. It is generally believed that the more training data there is, the more effective the deep learning model will be. Deep learning models have achieved significant progress which exceeds human performance in many areas such as face recognition, object classification and person re-identification.
In the Semantic Indexing stream, Australian researchers are striving to best utilise the strength of deep learning to benefit law enforcement in society. In the scenario described at the beginning of this post, extensive research and development has been conducted to train highly discriminative models to detect faces and pedestrians from cluttered scenes, with the existence of visual variances in viewpoint, camera resolution, rotation, occlusion, etc. To fully exploit the abundant data contained in surveillance videos, temporal information is seamlessly integrated with images, so that the nuances in visual appearance are captured accurately.
The researchers are also building deep learning models to differentiate various faces and pedestrians. In the models, visual similarities are provided by annotation experts as training data. The model pulls similar samples while pushing away dissimilar samples. In this manner, models are capable of telling whether a current image/language description can match a person-of-interest. Usually, this process is accelerated by powerful GPUs, through which the verification process is performed in real-time.
Not limited to Australia, many researchers from both academia and industry all over the world have been trying to apply deep learning to fight crime in different directions. These directions include intelligent crime investigators, missing person searches and firearm identification.
Deep learning is currently making increasing progress in the law enforcement community by bridging the gap between large-scale low-level training data and the high-level recognition goals. In the future, much effort will be made on learning end-to-end visual recognition systems that connect the essentials in object detection and recognition.